线性代数:向量空间-子空间
11 May 2017The review of the key ideas are from chapter 3 of Introduction to Linear Algebra, Book of Gilbert Strang, editon 4th.
- Spaces of vectors
- The nullspace of A: solving \(Ax=0\).
- The rank and the row reduced form
- The complete solution to \(Ax=b\)
- Independence, Basis, and Dimension
- Dimension of the Four Subspaces
1.Spaces of vectors
1.0 Key ideas
- \(R^n\) contains all column vectors with \(n\) real components.
- \(M\)(2 by 2 matrices) and \(F\)(functions) and \(Z\)(zero vector alone) are vector spaces.
- A subspace containing \(v\) and \(w\) must contain all their combinations \(cv\ + dw\).
- The combinations of the columns of \(A\) form the column space \(C(A)\). Then the column space is “spanned” by the columns(由列向量线性组合而成的空间 then spanned.).
- \(Ax=b\) has a solution exactly when \(b\) is in the column space of \(A\).
1.1 Spaces of Vectors
DEFINITION The space \(R^n\) consists all column vectors \(v\) with n components. (The components of \(v\) are real(R) numbers.)
In the definition of a vector space, vector addition \(x+y\) and scalar multiplication \(cx\) must obey the following eight rules:
- \[x + y = y + x\]
- \[x + (y + z) = (x + y) + z\]
- There is a unique “zero vector” such that \(x + 0 = x\) for all \(x\)(0 is zero vector)
- For each \(x\) there is a unique vector \(-x\) that \(x + (-x) = 0\)
- 1 times \(x\) equals \(x\)
- \[(c_1c_2)x=c_1(c_2x)\]
- \[c(x + y) = cx + cy\]
- \[(c_1 + c_2)x = c_1x + c_2x\]
上面8条规则离不开的是向量的加法和数乘(其中 \(c\) 是系数, \(x,y\)是向量空间中的向量).所有向量空间必须满足以上8项规则. 最基本的还是向量加法和数乘. 向量空间中的向量作以上加法和数乘后所得到的向量必定在向量空间内.若不满足8规,这不是向量空间.
符号 | 含义 |
---|---|
\(M\) | The vector space of all real 2 by 2 matrices |
\(F\) | The vector space of all real functions \(f(x)\) |
\(Z\) | The vector space that consists only of a zero vector |
1.2 Subspaces
DEFINITION A subspace of a vector space is a set of vectors(including 0) that satisfies two requirements: If \(v\) and \(w\) are vectors in the subspace and \(c\) is an scalar, then
- \(v+w\) is in the subspace.
- \(cv\) is in the subspace.
已经确定一个向量空间之后(8规满足), 子空间的判定只需要上面两个规则.
In short, all linear combinations stay in the subspace.
- Facts:
- Every subspace contain the zero vector.
- Lines through the origin are also subspaces.
A subspace containing \(v\) and \(w\) must contain all linear combinations \(cv + dw\).
1.3 The Column Space of A
Remember: \(Ax\) is a combination of the columns of \(A\). So to sovle \(Ax=b\) is to express \(b\) as a combination of the columns. The right side \(b\) has to be in the column space produce by \(A\) on the left side, or no solution.
DEFINITION: The column space consists of all linear combinations of the columns. The combinations are all possibble vectors \(Ax\). They fill the column space \(C(A)\).
看, 从向量空间和线性组合理解线性方程组\(Ax=b\), 是不是更容易理解啦! 想要方程有解, 那么\(b\)必须在\(A\)的列向量空间中.
2.The nullspace of A: solving \(Ax=0\).
2.0 Key ideas
- The nullspace \(N(A)\) is a subspace of \(R^{n}\). It contains all solutions to \(Ax=0\).
- Elimination produces an echelon matrix \(U\), and then a row reduced \(R\), with pivot columns and free columns.
- Every free column of \(U\) or \(R\) leads to a special solution. The free variable equals 1 and the other free variables equals 0. Back substitution solves \(Ax=0\).
- The complete solution to \(Ax=0\) is a combination of the special solutions.
- If \(n > m\) then \(A\) has at least one column without pivots, giving a special solution. So there are nonzero vectors \(x\) in the nullspace of this rectangular \(A\).
2.1 Nullspace of \(Ax=0\)
The nullspace of \(A\) consists of all solutions to \(Ax=0\). These vectors \(x\) are in \(R^n\). The nullspace containing all solution of \(Ax=0\) is denoted by \(N(A)\).
The nullspace consists of all combinations of the special solutions.
The free components correspond to columns without pivots. The special choice(one or zero) is only for the free variables.
我们来看看下面三个矩阵:
\[A = \begin{bmatrix} 1 & 2 \\ 3 & 8 \end{bmatrix}, B = \begin{bmatrix}A \\ 2A \end{bmatrix} = \begin{bmatrix}1 & 2 \\ 3 & 8 \\ 2 & 4 \\ 6 & 16\end{bmatrix}, C = \begin{bmatrix} A \\ 2A \end{bmatrix}= \begin{bmatrix} 1 & 2 & 2 & 4 \\ 3 & 8 & 6 & 16 \end{bmatrix}.\]对他们消元:
\(A \Rightarrow \begin{bmatrix} 1 & 2 \\ 0 & 2 \end{bmatrix}\), \(C \Rightarrow U = \begin{bmatrix} 1 & 2 & 2 & 4 \\ 0 & 2 & 0 & 4 \end{bmatrix} \Rightarrow R = \begin{bmatrix} 1 & 0 & 2 & 0 \\ 0 & 1 & 0 & 2 \end{bmatrix}\), C消元之后, 前两列为 pivot columns, 后两列为 free columns. 那么特解 special solution 就容易得到啦. 怎么得到, 自己去想(自由变量分别设为1,0等).
Once we have the special solutions, we have the whole nullspace.
2.2 Echelon Matrices
\(U = \begin{bmatrix} p & x & x & x & x & x & x \\0 & p & x & x & x & x & x \\0 & 0 & 0 & 0 & 0 & p & x \\0 & 0 & 0 & 0 & 0 & 0 & 0 \end{bmatrix}\).
Three pivot variables \(x_1, x_2, x_6\).
Four free variables \(x_3, x_4, x_5, x_7\).
Four special solutions in \(N(U)\)
\(A\) is \(m\) by \(n\) matrix. If \(n>m\), there must be free variables, then, equation \(Ax=0\) must have nonzero vectors in its nullspace.
2.3 The Reduced Row Echelon Matrix R
\(U = \begin{bmatrix} 1 & 1 & 2 & 3 \\ 0 & 0 & 4 & 4 \\ 0 & 0 & 0 & 0 \end{bmatrix} \Rightarrow R=rref(A) = \begin{bmatrix} 1 & 1 & 0 & 1 \\ 0 & 0 & 1 & 1 \\ 0 & 0 & 0 & 0\end{bmatrix}\).
3.The rank and the row reduced form
3.0 Key ideas
- The rank \(r\) of \(A\) is the number of pivots(which are 1’s in \(R=rref(A)\)).
- The \(r\) pivot columns of \(A\) and \(R\) are in the same list pivcol.
- Those \(r\) pivot columns are not combinations of earlier columns.
- The \(n-r\) free columns are combinations of earlier columns(pivot columns).
- Those combinations (using \(-F\) taken from \(R\)) give the \(n-r\) special solutions to \(Ax=0\) and \(Rx=0\). They are the \(n-r\) columns of the nullspace matrix \(N\).
3.1 Rank and the row reduced form
DEFINITION: The rank of \(A\) is the number of pivots. This number is \(r\).
Every “free column” is a combination of earlier pivot columns.
Example:
\[A = \begin{bmatrix}1 & 1 & 2 & 4 \\ 1 & 2 & 2 & 5 \\ 1 & 3 & 2 & 6\end{bmatrix} \Rightarrow U = \begin{bmatrix} 1 & 1 & 2 & 4 \\ 0 & 1 & 0 & 1 \\ 0 & 0 & 0 & 0\end{bmatrix} \Rightarrow R = \begin{bmatrix} 1 & 0 & 2 & 3 \\ 0 & 1 & 0 & 1 \\ 0 & 0 & 0 & 0 \end{bmatrix}\]“Pivot column”: 有主元 pivot 的那一列.
Rank one matrix:
\(A = \begin{bmatrix} 1 & 3 & 10 \\ 2 & 6 & 20 \\ 3 & 9 & 30 \end{bmatrix} \Rightarrow R = \begin{bmatrix} 1 & 3 & 10 \\ 0 & 0 & 0 \\ 0 & 0 & 0 \end{bmatrix}\).
Pivot row [1 3 10]
Pivot variable \(x_1\)
Free variables \(x_2, x_3\)
special solutions: \(s_1 = \begin{bmatrix} -3 \\ 1 \\ 0 \end{bmatrix}, s_2 = \begin{bmatrix} -10 \\ 0 \\ 1 \end{bmatrix}\).
The rank \(r\) is the “dimension” of the column space. So the number \(n-r\) is the “dimension” of the Nullspace of \(A\).
3.2 The Pivot Columns
The pivot columns are not combinations of earlier columns. The free columns are combinations of earlier columns. These combinations are the special solutions.
3.3 The Special Solutions
\(Ax=0\) has \(r\) pivots and \(n-r\) free variables: \(n\) columns minus \(r\) pivot columns. The nullspace matrix \(N\) contains the \(n-r\) special solutions. Then \(AN=0\).
\(Ax=0\) has \(r\) independent equations so it has \(n-r\) independent solutions.
4.The complete solution to \(Ax=b\)
4.0 Key Ideas
- The rank \(r\) is the number of pivots. The matrix \(R\) has \(m-r\) zero rows.
- \(Ax=b\) is solvable if and only if the last \(m-r\) equations reduce to \(0=0\).
- One paricular solution \(x_p\) has all free variables equal to zero.[Please check it. Noting]
- The pivot variables are determined after the free variables are chosen.
- Full column rank \(r=n\) means no free variables: one solution or none.
- Full row rank \(r=m\) means one solution if \(m=n\) or infinitely many if \(m<n\).
4.1 Intro
解方程 \(Ax=b\) 和 \(Ax=0\) 类似, 行变换得到 \(Rx=d\).
\[\begin{bmatrix} 1 & 3 & 0 & 2 \\ 0 & 0 & 1 & 4 \\ 1 & 3 & 1 & 6 \end{bmatrix} \begin{bmatrix} x_1 \\ x_2 \\ x_3 \\ x_4 \end{bmatrix} \Rightarrow \begin{bmatrix} 1 & 3 & 0 & 2 & 1 \\ 0 & 0 & 1 & 4 & 6 \\ 1 & 3 & 1 & 6 & 0 \end{bmatrix} = \begin{bmatrix} R & d \end{bmatrix}\]以上方程组有无数解.
Here are the same augmented matrices for a general \(b = (b_1, b_2, b_3)\):
\[\begin{bmatrix} A & b \end{bmatrix} = \begin{bmatrix} 1 & 3 & 0 & 2 & b_1 \\ 0 & 0 & 1 & 4 & b_2 \\ 1 & 3 & 1 & 6 & b_3 \end{bmatrix} \Rightarrow \begin{bmatrix} 1 & 3 & 0 & 2 & b_1 \\ 0 & 0 & 1 & 4 & b_2 \\ 0 & 0 & 0 & 0 & b_3-b_1 -b_2\end{bmatrix} = \begin{bmatrix} R & d \end{bmatrix}\]So if \(b_3-b_1-b_2=0\), then \(0=0\), \(Ax=b\) have many solutions.
4.2 One Particular Solution
如何得到上面方程组的特解呢? 将自由变量设为 \(x_2 = x_4 = 0\), 再解方程, 我们就得到\(x_1 = 1, x_3 = 6\), 得到特解: \(x_p = (1, 0, 6, 0)\).
Free variables = zero, pivot variables from d. WOW, 这个总结太棒了.
\(Rx_p = \begin{bmatrix} 1 & 3 & 0 & 2 \\ 0 & 0 & 1 & 4 \\ 0 & 0 & 0 & 0 \end{bmatrix} \begin{bmatrix} \bf 1 \\ 0 \\ \bf 6 \\ 0 \end{bmatrix} = \begin{bmatrix} \bf 1 \\ \bf 6 \\ 0 \end{bmatrix}\) Pivot variables 1, 6. Free variables 0, 0.
\(x_{particular}\): The particular solution solves \(Ax_p = b\).
\(x_{nullspace}\): The \(n-r\) special solutions solve \(Ax_n = 0\).
通解: 特解和0解集合.
\[m = n = r, \bf{x = x_p + x_n = A^{-1}b + 0}.\]Complete solution one \(x_p\) many \(x_n\) : \(x = x_p + x_n = \begin{bmatrix} 1 \\ 0 \\ 6 \\ 0 \end{bmatrix} + x_2 \begin{bmatrix} -3 \\ 1 \\ 0 \\ 0 \end{bmatrix} + x_4 \begin{bmatrix} -2 \\ 0 \\ -4 \\ 1 \end{bmatrix}\)
列满秩矩阵\(A\): (\(m>n=r\))
Full column rank \(R = \begin{bmatrix} I \\ 0 \end{bmatrix} = \begin{bmatrix} n\ by\ n\ identity\ matrix \\ m-n\ rows\ of\ zeros \end{bmatrix}\)
列满秩矩阵其属性:
- All columns of \(A\) are pivot columns.
- There are no free variables or special solutions.
- The nullspace \(N(A)\) contains only the zero vector \(\bf{x=0}\).
- If \(A \bf x = \bf b\) has a solution (it might not) then it has only one solution. This \(A\) has independent columns.
列满秩矩阵\(A\), 其方程组\(A \bf x = \bf b\) 有一个解 或 无解. 这就要看 \(\bf b\) 若消元后, 增广矩阵 \(m-r\) 行 都为零, 则只有一个解, 若不为0, 则无解.
4.3 The Complete Solution
另外一种情况, 就是方程组 \(A \bf x = \bf b\) 只有一个解, 或 无数个解. –> full row rank matrix. (\(r=m\))
Every matrix \(A\) with full row rank (\(r=m\)) has all these properties:
- All rows have pivots, and \(R\) has no zero rows.
- \(A \bf x = \bf b\) has a solution for every right side \(\bf b\).
- The column space is the whole space \(\bf R^{m}\).
- There are \(n - r = n - m\) special solutions in the nullspace of \(A\).
The four possibilities for linear equations depend on the rank \(r\):
rows \(m\) | columns \(n\) | Descriptions | Solutions |
---|---|---|---|
\(r=m\) & | \(r=n\) | Square and invertible | \(Ax=b\) has 1 solution |
\(r=m\) & | \(r<n\) | Short and wide | \(Ax=b\) has \(\infty\) solutions |
\(r<m\) & | \(r=n\) | Tall and thin | \(Ax=b\) has 0 or 1 solutions |
\(r<m\) & | \(r<n\) | Not full rank | \(Ax=b\) has 0 or \(\infty\) solutions |
5.Independence, Basis, and Dimension
5.0 Key ideas
- The columns of \(A\) are independent if \(\bf {x=0}\) is the only solution to \(\bf {Ax=0}\).
- The vectors \(v_1, ..., v_r\) span a space if their combinations fill that space.
- A basis consists of linearly independent vectors that span the space. Every vector in the space is a unique combination of the basis vectors.
- All bases for a space have the same number of vectors. This number of vectors in a basis is the dimension of the space.
- The pivot columns are one basis for the column space. The dimension is \(\bf r\).
The true dimension of the column space is the rank \(r\).
A basis: independent vectors that “span the space”.
Every vector in the space is a unique combination of the basis vectors.
5.1 Linear Independence
线性独立:
DEFINITION The columns of \(A\) are linearly independent when the only solution to \(\bf Ax=0\) is \(\bf x=0\). No other combination \(Ax\) of the columns gives the zero vecotrs.
即: 如果 \(\bf Ax=0\) 只有0解, 那么, \(A\)的各列线性独立.(When the nullspace \(N(A)\) contains only the zero vector.)
DEFINITION The sequence of vectors \(v_1, v_2, ..., v_n\) is linearly independent if the only combination that gives the zero vector is \(0v_1 + 0v_2 + \cdots + 0v_n\).
Linear Independence |
---|
\(x_1v_1 + x_2v_2 + \cdots + x_nv_n = \bf 0\) only happens when all \(x\)’s are zero |
Full column rank The columns of \(A\) are independent exactly when the rank is \(r=n\). There are n pivots and no free variables. Only \(\bf x=0\) is in the nullspace.
Any set of n vectors in \(R^m\) must be linearly dependent if \(n>m\).
举个例子: 矩阵\(A_{5,7}\), 5 by 7 matrix, 它的行向量必定不是线性独立. Any seven vectors from \(R^5\) are dependent.
5.2 Vectors that Span a Subspace
The first subspace in the book was the column space. The column space consists of all combinations \(Ax\) of the columns.
DEFINITION A set of vectors span a space if their linear combinations fill the space.
重要一点: span 莫要与 dependence 混淆.
The columns of a matrix span its column space. They might be dependent.
向量 span 一个空间, 这些向量不一定线性独立. 看具体情况.
Example:
\(v_1 = \begin{bmatrix} 1 \\ 0 \end{bmatrix}, v_2 = \begin{bmatrix} 0 \\ 1 \end{bmatrix}\) span the full 2-dimensional space \(R^2\).
\(v_1 = \begin{bmatrix} 1 \\ 0 \end{bmatrix}, v_2 = \begin{bmatrix} 0 \\ 1 \end{bmatrix}, v_3 = \begin{bmatrix} 4 \\ 7\end{bmatrix}\) also span the full space \(R^2\).
\(w_1=\begin{bmatrix} 1 \\ 1 \end{bmatrix}, w_2 = \begin{bmatrix} -1 \\ -1 \end{bmatrix}\) only span a line in \(R^2\). so does \(w_1\) by itself.
New subspace: The combinations of the rows produce the “row space”.
DEFINITION The row space of a matrix is the subspace of \(R^n\) spanned by the rows. The row space of \(A\) is \(C(A^T)\). It is the column space of \(A^T\)
5.3 A Basis for a Vector Space
Two vectors can’t span all of \(R^3\), even if they are independent. Four vectors can’t be independent, even if they span \(R^3\). We want enough independent vectors to span the space(and not more). A “basis” is just right.
DEFINITION A basis for a vector space is a sequence of vectors with two properties: The basis vectors are linearly independent and they span the space.
There is one and only one way to write \(v\) as a combination of the basis vectors.
\[A=\begin{bmatrix} 1 & 0 & 0 \\ 1 & 1 & 0 \\ 1 & 1 & 1\end{bmatrix}\]The vectors \(v_1, ..., v_n\) are a basis for \(R^n\) exactly when they are the columns of an n by n invertible matrix. The \(R^n\) has infinitely many different bases.
The pivot columns of A are a basis for it column space. The pivot rows of \(A\) are a basis for its row space. So are the pivot rows of its echelon form \(R\).
5.4 Dimension of a Vector Space
DEFINITION The dimension of a space is the number of vectors in every basis.
It is the dimension of the column space that equals the rank of the matrix r.
5.5 Bases for Matrix Spaces and Function Spaces
Matrix spaces The vector space \(M\) contains all 2 by 2 matrices. Its dimension is 4.
One basis is \(A_1, A_2, A_3, A_4 = \begin{bmatrix} 1 & 0 \\ 0 & 0 \end{bmatrix}, \begin{bmatrix} 0 & 1 \\ 0 & 0 \end{bmatrix}, \begin{bmatrix} 0 & 0 \\ 1 & 0 \end{bmatrix}, \begin{bmatrix} 0 & 0 \\ 0 & 1 \end{bmatrix}.\)
Every \(A\) combines the basis matrices \(c_1A_1 + c_2A_2 + c_3A_3 + c_4A_4 = \begin{bmatrix} c_1 & c_2 \\ c_3 & c_4\end{bmatrix} = A.\)
\(A\) is zero only if the c’s are all zero – this prove Independence of \(A_1, A_2, A_3, A_4.\)
\(n\) by \(n\) 矩阵空间有 \(n^2\) 个 basis matrices.
- The dimension of the whole \(n\) by \(n\) matrix space is \(n^2\).
- The dimension of the subspace of upper triangular matrices is \(\frac{1}{2} n^2 + \frac{1}{2} n\).
- The dimension of the subspace of diagonal matrices is \(n\).
- The dimension of the subspace symmetric matrices is \(\frac{1}{2} n^2 + \frac{1}{2} n\).
Function spaces:
- \(y^{"} = 0\) is solved by any linear function \(y = cx + d\).
- \(y^{"} = -y\) is solved by any combination \(y = c \sin x + d \cos x\).
- \(y^{"} = y\) is solved by any combination \(y = ce^{x} = de^{-x}\).
The empty set (containing no vectors) is a basis for Z(space that contains only the zero vector, the dimension of this space is zero).
6.Dimension of the Four Subspaces
6.0 Key ideas
- The \(r\) pivot rows of \(R\) are a basis for the row spaces of \(R\) and \(A\) (same space).
- The \(r\) pivot columns of \(A(!)\) are a basis for its column space.
- The \(n-r\) special solutions are a basis for the nullspaces of \(A\) and \(R\)(same space).
- The last \(m-r\) rows of \(I\) are a basis for the left nullspace of \(R\).
- The last \(m-r\) rows of \(E\) are a basis for the left nullspace of \(A\).
Main theorem: rank and dimension. The rank of a matrix is the number of pivots. The dimension of a subspace is the number of vectors in a basis.
The rank of \(A_{m,n}\) reveals the dimensons of all four fundamental subspaces:
- The row space is \(C(A^T)\), a subspace of \(R^n\).
- The column space is \(C(A)\), a subspace of \(R^m\).
- The nullspace is \(N(A)\), a subspace of \(R^n\).
- The left nullspace is \(N(A^T)\), a subspace of \(R^m\). (new space.)
For the left nullspace we solve \(A^Ty=0\)—that system is n by m. This is the nullspace of \(A^T\). \(A^Ty=0 \rightarrow y^{T}A=\bf 0^T\).
Facts
The row space and column space have the same dimension \(r\)(the rank of the matrix).
\(N(A), N(A^T)\) have dimension \(n-r\), \(m-r\), to make up the full \(n\) and \(m\).
6.1 The Four Subspaces for R
The echelon matrix \(R_{m,n}\):
\[R=\begin{bmatrix} 1 & 3 & 5 & 0 & 7 \\ 0 & 0 & 0 & 1 & 2 \\ 0 & 0 & 0 & 0 & 0 \end{bmatrix}\]- \(m=3, n=5, r=2\)
- pivot rows 1, 2
- pivot columns 1, 4
So:
- The row space of \(R\) has dimension 2, matching the rank.
- The dimension of the row space is the rank \(r\). The nonzero rows of \(R\) form a basis.
- The column space of \(R\) also has dimension \(r=2\).
- The dimension of the column space is the rank \(r\). The pivot columns form a basis.
- The nullspace has dimension \(n-r=5-2\). There are \(n-r=3\) free variables. Here \(x_2, x_3, x_5\) are free (no pivots in those columns). They yield the three special solutions to \(Rx=0\). Set a free variable to 1, and solve for \(x_1, x_4\).
- The nullspace has dimension \(n-r\). The special solutions form a basis.
- The nullspace of \(R^T\) (left nullspace of \(R\)) has dimension \(m-r = 3-2 = 1\).
以上, 看多了, 习题做多了, 自然而然就知道咯.
6.2 The Four Subspace for A
一图解疑. 自己琢磨.
6.3 Matrices of Rank One
Every rank one matrix has the special form \(A=uv^T=\)column times row.
@Anifacc
2017-05-16

人生苦短, 为欢几何.
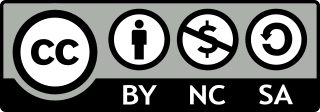