线性代数:正交
17 May 2017The review of the key ideas are from chapter 4 of Introduction to Linear Algebra, Book of Gilbert Strang, editon 4th.
- Orthogonality of the Four Subspaces
- Projections
- Least Square Approximations
- Orthogonal Bases and Gram-Schmidt
1. Orthogonality of the Four Subspaces
1.0 Key ideas
- Subspaces \(\bf V\) and \(\bf W\) are orthogonal if every \(v\) in \(\bf V\) is orthogonal to every \(w\) in \(\bf W\).
- \(\bf V , \bf W\) are “orthogonal complements” if \(\bf W\) contains all vectors perpendicular to \(\bf V\)(and vice versa). Inside \(R^n\), the dimensions of complements \(\bf V\) and \(\bf W\) add to \(n\).
- The nullspace \(N(A)\) and the row space \(C(A^T)\) are orthogonal complements, from \(Ax=0\). Similarly \(N(A^T)\) and \(C(A)\) are orthogonal complements.
- Any \(n\) independent vectors in \(R^n\) will span \(R^n\).
- Every \(x\) in \(R^n\) has s nullspace component \(x_n\) and a row space component \(x_r\).
1.1 Introduction
Orthogonal vectors \(v^T w = 0\) and \(\parallel v \parallel ^2 + \parallel w \parallel ^2 = \parallel v + w \parallel ^2\).
\(Ax\):
- At the first level: this is only numbers
- At the second level: a combination of column vectors
- At the third level: subspaces
The row space is perpendicular to the nullspace of \(A\): \(Ax=0\).
The column space is perpendicular to the nullspace of \(A^T\): \(A^Tx=0\), the row space of \(A^T\) is the column space of \(A\). When \(b\) is outside the column space – when we want to solve \(Ax=b\) and can’t do it – then this nullspace of \(A^T\) comes into its own. It contains the error \(e=b-Ax\) in the “least-squares” solution.
DEFINITION Two subspaces \(V, W\) of a vector space are orthogonal if every vector \(v\) in \(V\) is perpendicular to every vector \(w\) in \(W\).
Orthogonal subspaces \(v^T w = 0\) for all \(v\) in \(V\) and all \(w\) in \(W\).
The row space \(C(A^T)\) and nullspace \(N(A)\) are orthogonal subspaces inside \(R^n\).
The left nullspace \(N(A^T)\) and the column space \(C(A)\) are orthogonal in \(R^m\).
1.2 Orthogonal Complements
DEFINITION The orthogonal complement of a subspace \(V\) contains every vector that is perpendicular to \(V\). This orthogonal subspace is denoted by \(V^{\perp}\) (“\(V\) perp”).
Fundamental Theorem of Linear Algebra, Part 2 |
---|
\(N(A)\) is the orthogonal complement of the row space \(C(A^T)\)(in \(R^T\)) |
*\(N(A^T)\) is the orthogonal complement of the column space \(C(A)\) (in \(R^m\)) ** |
Part 1 gave the dimensions of the subpaces. Part 2 gives the 90° angles between them.
1.3 Combining Bases from Subspaces
Any n independent vectors in \(R^n\) must span \(R^N\). So they are a basis. Any n vectors that span \(R^n\) must be independent. So they are a basis.
\(A_{n,n}\):
If the n columns of \(A\) are independent, they span \(R^n\). So \(Ax=b\) is solvable. If the n columns span \(R^n\), they are independent. So \(Ax=b\) has only one solution.
怎么就觉得上面的叙述是一样的, 看不出多大差异, 那么到底有没有差别呢?
2. Projections
2.0 Key Ideas
- The projection of \(b\) onto the line through \(a\) is \(p=a \widehat x = a(a^T b/a^T a)\).
- The rank one projection matrix \(P= aa^T/a^Ta\) multiplies \(b\) to produce \(p\).
- Projecting \(b\) onto a subspace leave \(e = b - p\) perpendicular to the subspace.
- When \(A\) has full rank \(n\), the equation \(A^T A \widehat x = A^T b\) leads to \(\widehat x\) and \(p = A \widehat x\).
- The projection matrix \(P = A(A^T A)^{-1} A^T\) has \(P^T=P\) and \(P^2=P\).
2.1 Introduction
极简入手:
- What are the projections of \(b=(2, 3, 4)\) onto the \(z\) axis and the \(xy\) plane?
- What matrices produce those projections onto a line and a plane?
个例–>泛例:
第一个问题: 投影到z轴的向量为, \(p_1=(0,0,4)\), 投影到 \(xy\) 平面的向量为 \(p_2 = (2,3,4)\)
分别对应的投影矩阵为:
Onto the \(z\) axis: \(P_1 = \begin{bmatrix} 0 & 0 & 0 \\ 0 & 0 & 0 \\ 0 & 0 & 1 \end{bmatrix}\). Onto the \(xy\) plane \(P_2 = \begin{bmatrix} 1 & 0 & 0 \\ 0 & 1 & 0 \\ 0 & 0 & 0\end{bmatrix}\)
\(p_1 = P_1 b = \begin{bmatrix} 0 & 0 & 0 \\ 0 & 0 & 0 \\ 0 & 0 & 1 \end{bmatrix} \begin{bmatrix} x \\ y \\ z\end{bmatrix} = \begin{bmatrix} 0 \\ 0 \\ z \end{bmatrix}\).
\(p_2 = P_2 b = \begin{bmatrix} 1 & 0 & 0 \\ 0 & 1 & 0 \\ 0 & 0 & 0\end{bmatrix} \begin{bmatrix} x \\ y \\ 0 \end{bmatrix}\).
直观这两个投影向量:
- \(p_1, p_2\) are perpendicular
- The line and plane (subpaces) are orthogonal complements. The vectors gives \(p_1 + p_2 = b\). The matrices gives \(P_1 + P_2 = I\).
The best description of a subspace is a basis. We put the basis vectors into the columns of \(A\). Now we are projecting onto the column space of \(A\). Certainly the z axis is the column space of the 3 by 1 matrix \(A_1\). The \(xy\) plane is the column space of \(A_2\). That plane is also the column space of \(A_3\) (a subspace has mang bases):
\(A_1 =\begin{bmatrix} 0 \\ 0 \\ 1 \end{bmatrix}\) and \(A_2 =\begin{bmatrix} 1 & 0 \\ 0 & 1 \\ 0 & 0 \end{bmatrix}\) and \(A_3 = \begin{bmatrix} 1 & 2 \\ 2 & 3 \\ 0 & 0 \end{bmatrix}\)
Our problem is to project any \(b\) onto the column space of any \(m\) by \(n\) matrix. Start with a line (dimensions n = 1). The matrix A has only column. Call it \(\bf a\).
2.2 Projection Onto a Line
A line goes through the origin in the direction of \(\bf a = (a_1, a_2, \cdots, a_m)\). In figure 4-5, the line from \(\bf b\) to \(\bf p\) is perpendicular to the vector \(\bf a\).
Projecting \(\bf b\) onto \(\bf a\), error \(\bf e = \bf {b-\widehat x a}, \bf{a\cdot(b- \widehat x a)} = 0\), or \(\bf{a \cdot b - \widehat x a \cdot a} = 0 \rightarrow \bf{\widehat x = \frac{a \cdot b}{a \cdot a} = \frac{a^T b}{a^T a}}\).
So:
The projection of \(\bf b\) onto the line through \(\bf a\) is the vector \(\bf{p = \widehat x a = \frac{a^T b}{a^T a} a}\).
Special case 1: \(\bf {b = a}\).
Special case 2: \(\bf b\) is perpendicular to \(\bf a\).
Projection Matrix:
\(\bf{p = a \widehat x = a \frac{a^T b}{a^T a} = Pb}\). When the matrix is \(P = \bf{\frac{a a^T}{a^T a}}\).
Projecting a seconde time does not change anything, so \(P^2 = P\).
Note: the matrix \(I-P\) should be a projection too. \((I-P) \bf b = \bf{b-p} = \bf e\). When \(P\) projects onto one subspace, \(I-P\) projects onto the perpendicular subspace.
2.3 Projection Onto a Subspace
Start with \(n\) vectors \(\bf{a_1, a_2, \cdots, a_n}\) in \(R^m\). Assume that these \(\bf a\)’s are linear independent.
Problem: Find the combination \(\bf p = \widehat{x_1} \bf {a_1} + \cdots + \widehat{x_n} \bf{a_n}\) closet to a given vector \(\bf b\). Look in figure 4-5.
\(\bf{e = b - A \widehat{x}}\) is perpendicular to the subspace.
\(\matrix{a^{T}_{1} (b - A \widehat{x}) = 0 \\ \vdots \\ a^{T}_{n} (b - A \widehat{x}) = 0 }\) or \(\begin{bmatrix} ---a^{T}_{1}--- \\ \vdots \\ ---a^{T}_{n}--- \end{bmatrix} \begin{bmatrix} b - A \widehat{x} \end{bmatrix} = \begin{bmatrix} 0 \end{bmatrix}\).
\(\Rightarrow A^T(b-A \widehat{x})=0 \Rightarrow A^T A \widehat{x} = A^T b\).
\(p = A \widehat{x} = A(A^T A)^{-1} A^T b\).
project matrix: \(P = A(A^T A)^{-1} A^T\).
The key step is \(A^T(b-A \widehat{x})=0\). We used to geometry (\(\bf e\) is perpendicular to all the \(\bf a\)’s). Linear algebra gives this “normal equation” too, in a very way:
- Our subspace is the column space of \(A\).
- The error vector \(b-A \widehat{x}\) is perpendicular to the column space.
- Therefor \(b-A \widehat{x}\) is in the nullspace of A^T. This means \(A^T(b-A \widehat{x})=0\).
\(A^T A\) is invertible if and only if \(A\) has linearly independent columns.
\(A^T Ax=0\)
\(\rightarrow x^T A^T A x = 0\)
\(\rightarrow (Ax)^T(Ax)=0\) or
\(\parallel Ax \parallel ^ 2 = 0\)
When \(A\) has independent columns, \(A^T A\) is square, symmetric, and invertible.
3. Least Square Approximations
3.0 Key Ideas
- The least square solution \(\widehat x\) minimizes \(E = \parallel Ax-b \parallel^2\). This is the sum of squares of the errors in the \(m\) equations(\(m>n\)).
- The best \(\widehat x\) comes from the normal equations \(A^TA \widehat x = A^Tb\).
- To fit \(m\) points by a line \(b = C + Dt\), the normal eqautions give \(C\) and \(D\).
- The heights of the best line are \(p=(p_1, p_2, ..., p_m)\). The vertical distances to the data points are the errors \(e = (e_1, ..., e_m)\).
- If we try to fit \(m\) points by a combination of \(n<m\) functions, the \(m\) equations \(Ax=b\) are generally unsolvable. The \(n\) equations \(A^TA \widehat x = A^T b\) give the least squares solution – the combination with smallest MSE(mean square error).
3.1 Introduction
When \(Ax=b\) has no solution, multiply by \(A^T\) and solve \(A^T A \widehat x = A^Tb\).
3.2 Minimizing the Error
The least square solution \(\widehat x\) makes \(E = \parallel Ax-b \parallel ^ 2\) as small as possible.
Squared length for any \(x: \parallel Ax-b \parallel ^ 2 = \parallel Ax-p \parallel ^ 2 + \parallel e \parallel ^ 2\)
在上节正交的基础上可以得到: \(e=b-A \widehat x\) 与A中所有的列向量 \(a_n\) 垂直, 那么 \(A^T e =0 \rightarrow A^T (b - A \widehat x) = 0 \rightarrow A^T A \widehat x = A^T b\).
The partial derivatives of \(\parallel Ax-b \parallel ^ 2\) are zero when \(A^T A \widehat x = A^Tb\).
3.3 The Big Picture
自行体会.
3.4 Fitting a Straight Line
The closet line \(C+Dt\) has heights \(p_1, ..., p_m\) with errors \(e_1, ..., e_m\).
Solve \(A^TA \widehat X = A^T b\) for \(\widehat x = (C, \ D)\) . The errors are \(e_i = b_i - C -D t_i\).
解 \(A^TA \widehat X = A^T b\) 就可得到一元一次方程的系数。
拟合点为\((t_1, b_1), \cdot, (t_m, b_m)\), 则 \(A =\begin{bmatrix} 1 & t_1 \\ \vdots & \vdots \\ 1 & t_m \end{bmatrix}, b=\begin{bmatrix} b_1 \\ \vdots \\ b_m \end{bmatrix}\).
3.5 Fitting by Parabola
若是 \(C+Dt + Et^2\) 曲线拟合呢?
同理, 求解 解 \(A^TA \widehat X = A^T b\) 的方程系数.
拟合点为\((t_1, b_1), \cdot, (t_m, b_m)\), 则 \(A =\begin{bmatrix} 1 & t_1 & t_{1}^{2} \\ \vdots & \vdots & \vdots \\ 1 & t_m & t_{m}^{2}\end{bmatrix}, b=\begin{bmatrix} b_1 \\ \vdots \\ b_m \end{bmatrix}\).
4. Orthogonal Bases and Gram-Schmidt
4.0 Key Ideas
- If the orthonormal vectors \(q_1, \cdots , q_n\) are the columns of \(Q\), then \(q^{T}_{i}q_j=0\) and \(q^{T}_{i}q_i=0\) translate into \(Q^T Q=I\).
- If \(Q\) is square (an orthogonal matrix) then \(Q^T=Q^{-1}\): transpose = inverse.
- The length of \(Qx\) equals the length of \(x: \parallel{Qx}\parallel = \parallel{x}\parallel\).
- The projection onto the column space spanned by the \(q\)’s is \(P=QQ^T\).
- If \(Q\) is square then \(P=I\) and every \(b=q_1(q_1^Tb) + \cdots + q_n(q_n^Tb)\).
- Gram-Schmidt produces orthogonal vectors \(q_1, q_2, q_3\) from independent \(a, b, c\). In matrix form this is the Factorization \(A=QR=\)(orthogonal\(Q\))(triangular\(R\)).
4.1 Introduction
矩阵\(Q\)的列向量正交:
\(Q^T Q = \begin{bmatrix} ---q^{T}_{1} --- \\ ---q^{T}_{2} --- \\ \vdots \\ ---q^{T}_{n} --- \end{bmatrix} \begin{bmatrix} \mid & \mid & \cdots & \mid \\ q_1 & q_2 & \cdots & q_3 \\ \mid & \mid & \cdots & \mid \end{bmatrix} = I\).
When \(Q\) is square, \(Q^T Q=I\) means that \(Q^T=Q^{-1}\): transpose = inverse.
Examples:
Rotation: \(Q=\begin{bmatrix} \cos\theta & -\sin\theta \\ \sin\theta & \cos\theta \end{bmatrix}\)
Permutation: \(Q=\begin{bmatrix} 0 & 1 & 0 \\ 0 & 0 & 1 \\ 1 & 0 & 0 \end{bmatrix}\). Every Permutation matrix is an orthogonal matrix.
Reflection: If \(u\) is any unit vector, set \(Q=I-2uu^T\).
4.2 Projections Using Orthogonal Bases: Q Replaces A
Gram-Schmidt 基本原理就是投影得到正交向量矩阵.
第一步: \(A=a, B=b-\frac{A^Tb}{A^TA}A\)
第二步: \(C = c - \frac{A^Tc}{A^TA}A - \frac{B^Tc}{B^TB}B\).
(前提: 各向量\(a,b,c\)线性独立.)
4.3 The Factorization \(A=QR\)
\(\begin{bmatrix} \ & \ & \ \\ a & b & c \\ \ & \ & \ \end{bmatrix} = \begin{bmatrix} \ & \ & \ \\ q_1 & q_2 & q_3 \\ \ & \ & \ \end{bmatrix} \begin{bmatrix} q_1^T a & q_1^T b & q_1^T c \\ \ & q_2^Tb & q_2^Tc \\ \ & \ & q_3^Tc \end{bmatrix}\) or \(A=QR\).
\[A^TA=R^TQ^TQR=R^TR\]The least squares equation \(A^TA \widehat{x} = A^Tb\) simplifies to \(Rx=Q^Tb\):
Least squares \(R^TR \widehat{x} = R^TQ^Tb\) or \(R \widehat{x} = Q^Tb\) or \(\widehat{x} = R^{-1}Q^Tb\)
@Anifacc
2017-05-25

人生苦短, 为欢几何.
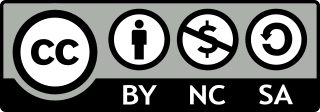